Artificial Intelligence and Ethical Challenges in Society
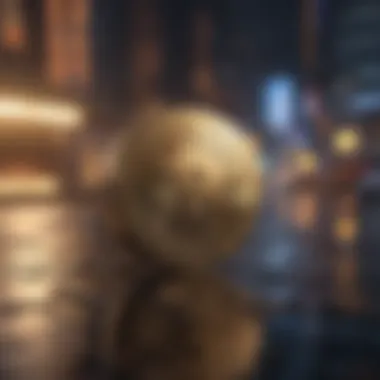
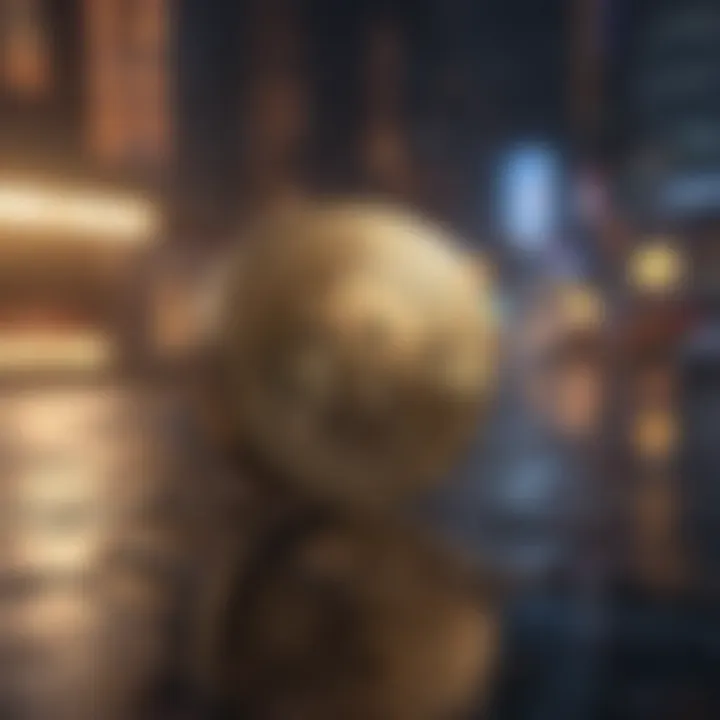
Intro
Artificial Intelligence (AI) has burrowed itself into the very fabric of our daily lives, influencing everything from how businesses operate to individual decision-making processes. Yet, while AI promises efficiency and innovation, it also brings a flurry of ethical concerns that demand meticulous examination. As we tread deeper into this digital age, understanding these ethical dilemmas becomes more critical than ever.
In this discourse, we shall underscore the major ethical issues entwined with the burgeoning capabilities of AI. It’s not simply about what AI can do or achieve but also what it should do and under whose oversight. The exploration is extended through various lenses—accountability, transparency, and inherent biases. By disentangling these woven threads, we intend to offer a clear roadmap for navigating the murky waters of AI ethics, ensuring that technological advancements align harmoniously with our core values.
The Relevance of Ethical Considerations in AI
When rolling out AI technologies, one must first ponder the implications they wield across society. Consider how algorithms decide what we see on social media, or how they influence hiring decisions in workplaces. The stakes are high; when these systems operate without oversight, they can perpetuate existing biases or introduce new inequities. As the saying goes, "with great power comes great responsibility." It is vital, therefore, to grasp the implications of accountability and governance that come with AI's rise.
Moreover, as AI systems continue to evolve, so too does the urgency to put boundaries around their capabilities, ensuring protection against unintended consequences. Thus, the need for comprehensive regulatory frameworks takes center stage—frameworks that not only foster innovation but also safeguard the fundamental human values we hold dear.
In this article, we will delve into these key themes and explore how they intersect through contemporary case studies, insights on data governance, the rise of autonomous machines, and the complexities surrounding bias in AI. Each section will paint a clearer picture of our digital landscape as we navigate these pressing ethical dilemmas.
To set the stage, we will first examine what accountability looks like within AI deployment. As the framework unfolds, it promises a comprehensive understanding of how we can channel the power of artificial intelligence responsibly.
Understanding Artificial Intelligence
In today's world, understanding artificial intelligence (AI) isn't just a nice-to-have—it's a necessity. As AI continues to weave itself into various aspects of our lives, from the mundane functions of our smartphones to complex algorithms guiding financial markets, grappling with its implications has become paramount. To navigate the ethical labyrinth that AI presents, one must first grasp what AI truly is and the breadth of its landscape.
Definition and Scope of AI
At its core, artificial intelligence refers to computer systems or machines designed to emulate human cognitive functions such as learning, reasoning, and problem-solving. While it may sound like a scene out of a science fiction flick, AI is present across diverse sectors.
AI encompasses a range of technologies, including:
- Machine Learning (ML): This subset allows machines to learn from data and improve over time without being explicitly programmed.
- Natural Language Processing (NLP): From chatbots to virtual assistants, this area enables machines to understand and interpret human language.
- Computer Vision: This involves the ability of a system to interpret and make decisions based on visual data from the world around it.
The importance of distinguishing between these facets lies in how they each present unique ethical considerations. For example, while ML can be trained to remove biases in decision-making, if the data itself is biased, the results will also reflect that.
Thus, defining and understanding the scope of AI is critical—not only to innovators but also to policymakers and educators. They need to recognize how these technologies will affect society at large.
Historical Development of AI Technologies
Tracing the roots of AI takes us back several decades. The concept traces back to the mid-20th century when pioneers like Alan Turing introduced the idea of machines that could simulate human intelligence. Fast forward to the present, and we witness exponential growth in AI capabilities, fueled by vast amounts of data, improved algorithms, and substantial computing power.
Several milestones highlight this evolution:
- The Turing Test: Proposed by Turing in 1950 as a criterion of intelligence. Can a machine exhibit behavior indistinguishable from that of a human?
- The Development of Expert Systems: In the 1970s and 1980s, AI took its first steps into the realm of specialized tasks such as medical diagnosis and financial forecasting.
- The Rise of Deep Learning: In the past decade, advances in neural networks have led to significant breakthroughs in tasks such as voice and image recognition.
This historical journey is essential in understanding the present landscape of AI, shedding light on past challenges and triumphs that inform current ethical considerations. As we analyze career implications, societal impacts, and regulatory measures, reflecting on the growth of AI technologies will provide a critical foundation for navigating the pulsing heart of ethical dilemmas in our technologically advanced society.
"The greatest challenge of AI is not its technology but rather our approach to the ethical dilemmas it presents."
Understanding AI is pivotal—both for unlocking its potential benefits and for understanding the ethical responsibilities we bear in implementing these technologies.
Ethical Frameworks in AI
The realm of artificial intelligence is not just about technology and algorithms; it's deeply intertwined with ethical considerations that shape societal values. Ethical frameworks in AI serve as the backbone of responsible innovation, guiding decisions that affect individuals and communities alike. These frameworks help to ensure that as we create intelligently capable machines, we also maintain respect for human rights and dignity.
Principles of AI Ethics
Ethics in AI often hinges on a set of core principles that encapsulate the essence of responsible technology use. Each principle underscores a unique aspect that directly enhances our approach to artificial intelligence.
Beneficence
The principle of beneficence revolves around fostering positive outcomes through AI systems. It emphasizes that AI should enhance human well-being, promoting good rather than harm. The hallmark of beneficence is its proactive nature, seeking to maximize the benefits of AI deployment.
The real beauty of this principle lies in its ability to push developers and organizations to prioritize societal good above all. However, the challenge comes in measuring what constitutes benefit. For instance, while one community may find automated healthcare reassuring, another might see it as encroaching on personal care. This dichotomy outlines the need for thoughtful assessment within the realm of beneficence.
Non-maleficence
Non-maleficence stands as a crucial counterpoint to beneficence. This principle asserts the imperative to avoid causing harm through AI. It echoes the timeless maxim: "do no harm." The key characteristic of non-maleficence is its cautionary stance, acting as a check on AI implementations that may endanger individuals or communities.
Now, consider a self-driving car. While it could reduce accidents in theory, what if the software makes a split-second decision that endangers pedestrians? This risk highlights the importance of robust testing and regulatory oversight to safeguard against unwanted consequences. Achieving non-maleficence demands rigorous analysis, as well as a commitment to transparency in design.
Justice
Justice in AI is all about fairness—ensuring equitable access and treatment across varied demographics. It's often characterized by its commitment to inclusivity. Inequities in AI outcomes can arise from biased data sets or disproportionate access to technology, leading to amplified societal disparities.
As such, adoptions that favor certain populations while marginalizing others contravene the principle of justice. Imagine an AI hiring system that inadvertently screens out candidates based on gender. The ramifications are profound, making justice not just a principle, but a necessity in creating systems that reflect societal values fairly. This principle serves as a reminder of our shared responsibility toward equitable technology dissemination.
Autonomy
Finally, autonomy underscores the need to respect individual freedom and choice in the face of AI advancement. This principle is significant because it advocates for enabling users to make informed decisions regarding AI interactions. For example, in the context of data privacy, autonomy involves allowing individuals to dictate how their personal information is utilized by AI systems.
This principle also champions the notion that AI should not unduly manipulate users or infringe on their decision-making capacity. However, navigating the balance between autonomy and algorithmic suggestions can be tricky. An overreliance on AI recommendations may unintentionally diminish personal agency. Thus, crafting AI systems that empower rather than constrict is essential for promoting autonomy.
Existing Ethical Guidelines and Standards
In the face of these principles, several ethical guidelines have emerged to navigate the complexities of AI usage. Organizations like the IEEE and the EU have spearheaded the development of comprehensive standards that aim to harmonize AI deployment with ethical considerations. These guidelines often include:
- Transparency: Ensuring that systems are understandable and their processes are clear.
- Accountability: Establishing frameworks for who is responsible in the event of failures.
- Inclusivity: Crafting systems that are fair and reachable for diverse populations.
These guidelines do not just remain theoretical; they actively shape policy-making, drive research, and influence corporate strategies. With ongoing discourse about AI's ethical ramifications, these standards are vital touchstones in our journey toward responsible AI.
Accountability and Responsibility in AI Systems
As artificial intelligence marches forward in its applications, the concepts of accountability and responsibility take center stage. The conversation surrounding AI isn't just about how smart machines are or what they can do; it's also about who bears the burden of their actions. In a world where decisions made by these systems can lead to significant consequences, understanding accountability becomes crucial for investors, educators, and analysts alike.
Defining Accountability in AI
Accountability, at its core, refers to the responsibility held by individuals or organizations for their actions or inactions. In the context of AI, it translates to determining who is responsible when AI systems produce outcomes that may be deemed harmful or unethical. Is it the programmer, the organization deploying the system, or the AI itself? This ambiguity can pose substantial challenges.
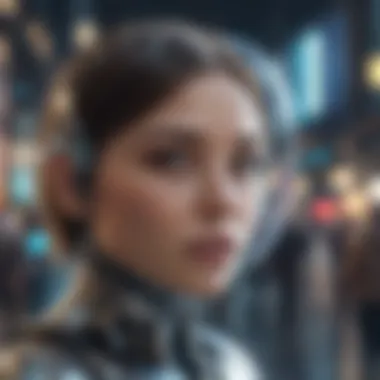
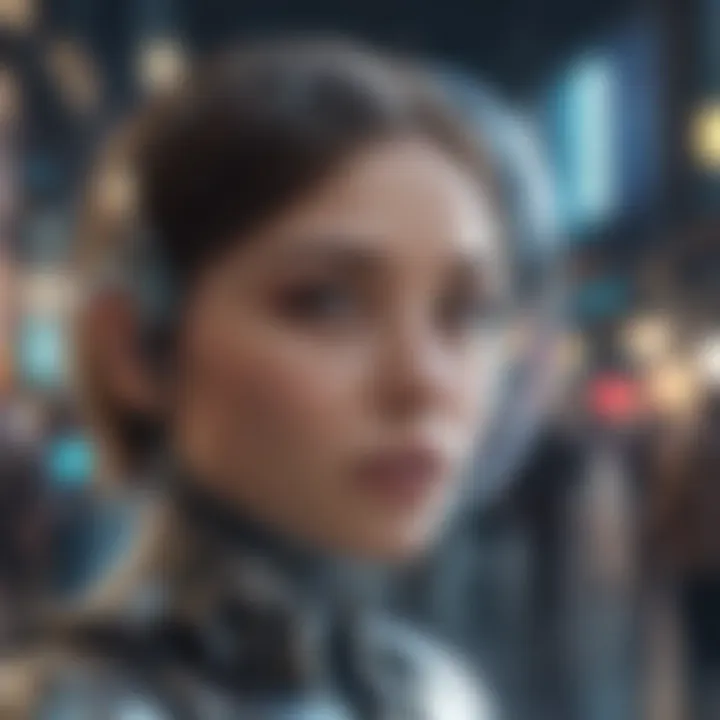
The primary elements involved in defining accountability in AI include:
- Ownership: Identifying who owns the data and algorithms is essential. Ownership influences whether the individual or corporation can be held liable.
- Governance: Establishing governance structures that support ethical AI use is critical. This can include oversight committees or third-party audits.
- Transparency: Ensuring that the decision-making processes of AI systems are transparent fosters accountability. If stakeholders understand how and why decisions are made, it’s easier to hold parties accountable.
An accountable AI system instills confidence among users and the public, which is vital for wider adoption of such technologies in various sectors.
Challenges in Assigning Responsibility
Despite the importance of establishing accountability in AI, numerous challenges hinder progress in this area. Key challenges include:
- Complexity of Systems: Many AI systems are black boxes, making it difficult to trace decisions back to specific algorithms or datasets. This lack of clarity complicates the assignment of responsibility.
- Distributed Responsibility: In many instances, responsibility can be shared among several actors, from developers to users. This diffusion of responsibility often leads to the blame game, resulting in no one person or group being held accountable.
- Rapid Innovation: The pace at which AI technology is advancing outstrips the development of legal frameworks. As a result, existing laws may not adequately address the nuances of AI—the gaps can lead to unregulated risk.
- Ethical Dilemmas: When an AI makes a mistake—say in a self-driving car accident—the ethical implications are heavy. How does one weigh harm done against the act of programming?
Accountability in AI is not merely a legal issue; it is also a moral one. Without accountability, the very fabric of trust in AI will unravel.
Navigating these challenges requires collaborative efforts among policymakers, engineers, and ethicists. Developing a well-defined accountability framework can assist in clarifying roles and responsibilities and ensure that AI deployment is done in a manner that respects human dignity and societal norms.
In summary, tackling accountability and responsibility within AI systems is a multi-faceted issue that has significant implications. As society embraces these technologies, ensuring that accountability is established and maintained will protect both individuals and communities from the pitfalls of unchecked systems.
Bias and Fairness in AI Algorithms
Bias and fairness in AI algorithms is a fundamental topic. It shapes how artificial intelligence systems operate, influencing their effectiveness and the ethical implications tied to their use. In a world where AI is integrated into various sectors, from healthcare to finance, the presence of bias can lead to unjust outcomes, eroding trust in these technologies. Thus, understanding bias not only assists in creating more equitable systems, but also ensures that these technologies align with societal values.
Understanding Algorithmic Bias
Algorithmic bias refers to the systematic and unfair discrimination against certain groups based on the data utilized to train AI systems. This phenomenon occurs when AI models perpetuate or amplify existing prejudices within their training datasets. It’s like baking a cake with rotten ingredients; the end result simply isn’t palatable, no matter how fancy the frosting.
Sources of Bias
Several sources contribute to algorithmic bias in AI. One major source is the data itself. If the training data reflects historical inequalities or is skewed towards a particular demographic, the AI will learn those biases. For instance, facial recognition systems have been shown to perform poorly with individuals from minority groups if predominantly trained on images of lighter-skinned people.
This key characteristic of data bias makes it a crucial consideration in the design and deployment phase of AI systems. The data can either be a bridge to equity or a barrier to fairness. Unique features of this source include the origins of the data—whether datasets are comprehensive and representative of all groups. If not managed carefully, they can lead to disadvantages, particularly in high-stakes scenarios like hiring processes or law enforcement algorithms.
Impacts of Bias
The impact of bias in AI is profound. It can lead to negative real-world consequences, such as discriminatory practices in hiring, lending, and law enforcement. When AI systems exhibit biased behavior, they may inadvertently reinforce social inequities, widening the gap between different groups.
One pivotal aspect of impacts of bias is its societal ripple effect. It may damage the credibility of AI technologies, stoking public fear and resistance towards their adoption. On the flip side, addressing these impacts proactively can enhance AI's perceived value, fostering public trust and acceptance. The unique challenge here is finding effective methods to measure and understand these impacts without feeling overwhelmed by the complexity of AI ethics.
Strategies to Mitigate Bias
To enhance fairness in AI algorithms, a multi-faceted approach is vital. Strategies may include auditing datasets for bias, employing diverse teams during development to provide broader perspectives, and adopting fairness-aware algorithms that recognize and adjust for inherent biases.
"The prudent approach to combatting bias starts during the data collection phase, where mindfulness can lead to significant prevention of future ethical dilemmas."
Incorporating continuous monitoring and feedback loops can further help in identifying biased outputs and correcting them post-deployment. By working consciously towards bias mitigation, the AI community stands to build systems that are not only more fair but also more reliable and beneficial to a diverse user base.
Transparency in AI Decision-Making
In the ever-evolving landscape of artificial intelligence, transparency in decision-making emerges as a cornerstone of ethical practices. This aspect is pivotal, not just for maintaining trust, but also for ensuring that AI technologies operate within acceptable moral boundaries. When algorithms and AI systems are perceived as opaque or inscrutable, it creates a fertile ground for skepticism and hesitance, especially among stakeholders affected by these technologies.
Understanding how decisions are made by AI systems can help mitigate fears or misconceptions. It guides the way we adopt and integrate AI into various sectors, ranging from healthcare to finance. An emphasis on transparency empowers users and practitioners to critically assess the actions of these systems and understand the rationale behind certain outputs or suggestions.
"In a world increasingly dominated by algorithms, transparency is not just beneficial; it's necessary for democratic accountability."
The importance of transparency in AI revolves around several key elements:
- Building Trust: Clear explanations of how AI systems reach their decisions foster trust among users.
- Accountability: When AI makes decisions, the ability to trace those decisions back to their sources creates a sense of responsibility.
- Better Collaboration: Stakeholders can collaborate more effectively when they understand the inner workings of AI tools.
- Error Identification: Knowing how AI reaches its conclusions helps in detecting and rectifying errors promptly.
Thus, the concept of transparency transcends a mere ethical obligation; it is intertwined with effective deployment and governance of AI.
Importance of Explainability
At its core, explainability refers to the capacity of an AI model to provide understandable descriptions of its outputs. In practice, this means users can grasp why a certain decision was made or a specific prediction was rendered.
In high-stakes domains like healthcare, where AI tools could dictate life-altering choices for patients, the need for explainability grows exponentially. It helps doctors, patients, and even regulatory bodies to trust that the AI is making sound recommendations based on clear, understandable logic.
However, the challenge remains significant. Many advanced models, such as deep learning neural networks, often function as "black boxes"—offering little to no insight into how inputs are transformed into outputs. The more complex the system, the less decipherable it becomes for casual observers. Therefore, the push for improving explainability not only enhances public confidence but also positions companies and organizations as responsible stewards of technology.
Techniques for Enhancing Transparency
Enhancing transparency in AI systems isn't just a theoretical pursuit; it requires practical strategies that can be systematically implemented. Here are some common techniques utilized to bring clarity:
- Documentation: Keeping comprehensive records of algorithm design, data sources, and model training processes helps track the evolution of AI systems.
- Model Visualization: Utilizing graphical representations to depict decision pathways can make complex models more approachable and easier to understand.
- User-Friendly Interfaces: Simplifying interaction interfaces so users can easily access information about the AI's decision-making process.
- Open-source Models: Leveraging open-source frameworks enables wider scrutiny, allowing developers and researchers to investigate and understand model workings collectively.
- Regular Audits: Frequent evaluations of algorithm outputs can facilitate ongoing assessments of fairness and accuracy.
Integrating these techniques fosters a culture of openness and trust, leading not just to a better understanding of AI systems but also to a commitment to ethical stewardship.
By prioritizing transparency, we move toward an era where AI serves as a collaborative partner in decision-making, rather than a distant oracle whose workings remain shrouded in mystery.
Privacy Concerns in AI Development
In the realm of artificial intelligence, the handling of personal data raises significant privacy issues. As AI systems increasingly rely on vast amounts of data to drive their learning processes, the risks associated with data privacy become more palpable. Protecting individuals' personal information is not just a legal requirement; it is a fundamental ethical obligation. The stakes are high as AI systems permeate our lives—be it through healthcare technology or social media algorithms—navigating these complexities is crucial.
Understanding privacy concerns in AI is vital because it underscores the need for control. Individuals must have confidence that their information is being handled appropriately and not exploited. In many ways, ensuring data privacy strengthens the relationship between society and technology.
Data Privacy Principles
Data privacy principles serve as a guiding framework for organizations developing AI technologies. These principles help safeguard personal information, fostering trust in AI systems. Key principles include:
- Consent: Users should have control over their data and provide explicit permission before it's collected or processed.
- Purpose Limitation: Data should only be collected for specific, legitimate reasons and not used for anything unrelated.
- Data Minimization: Organizations should limit the collection of data to what is necessary for their stated purpose.
- Accuracy: Data must be kept accurate and up to date to prevent harm that arises from incorrect information.
- Storage Limitation: Personal data should not be kept longer than necessary and should be securely deleted when it is no longer useful.
These principles function as the bedrock for responsible AI development, ensuring that privacy is not an afterthought but a priority in engineering methodologies.
Regulatory Frameworks Addressing Privacy
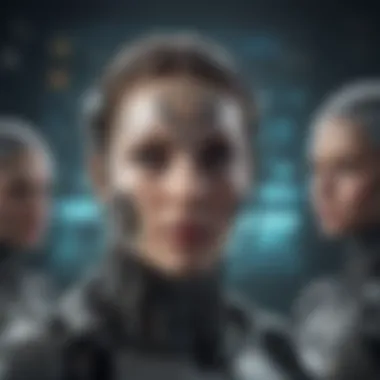
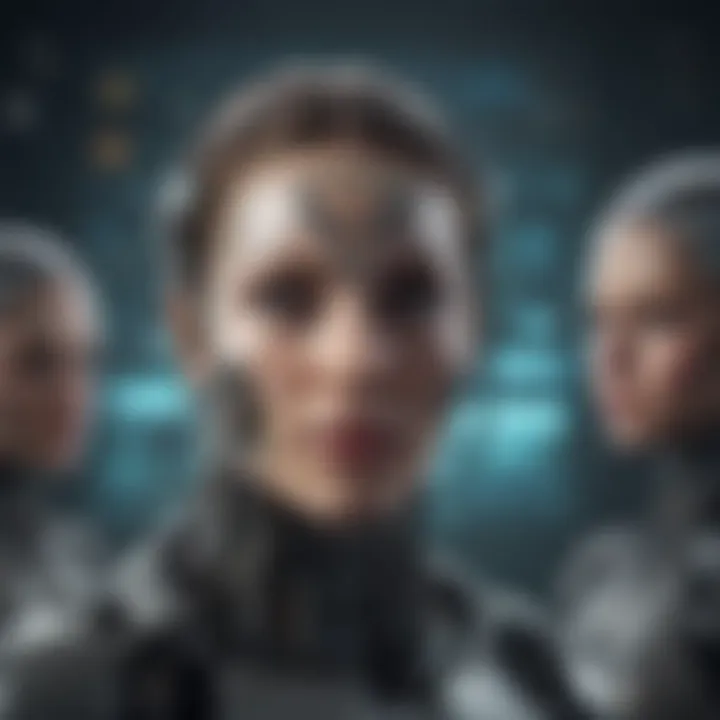
Regulatory frameworks are critical in addressing privacy concerns specific to AI. Various laws and regulations shape how organizations must approach data privacy. Notable regulations include:
- General Data Protection Regulation (GDPR): This European guideline offers robust protection for personal data, emphasizing users’ rights and imposing strict requirements on data collection.
- California Consumer Privacy Act (CCPA): Aimed at providing Californians greater control over their personal information, the CCPA establishes clear protocols for data usage and transparency.
- Health Insurance Portability and Accountability Act (HIPAA): Though primarily aimed at health information, HIPAA sets standards for the protection of sensitive patient data, impacting AI used in healthcare.
These frameworks are essential as they not only establish legal compliance but also promote ethical standards in AI systems. As the landscape of AI continues to evolve, adherence to these regulations is paramount for developers aiming to build trustworthy and ethical artificial intelligence.
"Privacy is not an option, and it shouldn't be the price we accept for just getting on the Internet." – Gary Kovacs
AI and Autonomy: Ethical Implications
The rise of artificial intelligence is much like a double-edged sword. On one side, we have remarkable advancements in technology promising to streamline processes, improve lives, and offer innovative solutions. On the other, serious ethical dilemmas loom, especially when we grapple with the concept of autonomy in AI systems. As we delve into this fascinating intersection, we will scrutinize moral considerations and real-world applications that underscore the importance of ethical implications in a world increasingly governed by autonomous machines.
Moral Considerations of Autonomous Systems
Autonomous systems, designed to operate independently with critical decision-making abilities, raise a host of moral questions. These machines, ranging from self-driving cars to robotic surgical systems, function without human intervention. This independence necessitates a deep dive into the responsibilities and ethical considerations tied to their actions.
First and foremost, there’s the concern of accountability. If a self-driving car gets into an accident, who bears the burden? The manufacturer? The software developer? Or the owner? This quandary highlights the complexity of assigning responsibility when machines have the capacity to act on their own. Adopting a clear framework for accountability is vital to prevent evasion of responsibility.
Another aspect revolves around the ethical programming of AI systems. Designers must embed moral values into algorithms. This raises profound questions: How do we decide which values to instill? Should AI prioritize human safety over property damage, or vice versa? There exists a delicate balance inherent in these decisions, necessitating a thorough examination of societal values and standards.
"The ethical debate surrounding AI and autonomy is not just about technology, but about what it means to be human in an automated world."
Moreover, autonomous systems must contend with biases that may be inherited from training data, which could lead to unfair treatment of certain groups. The moral implications here are severe, as unintended discrimination against individuals can result in grave consequences. Therefore, builders of autonomous systems must not only be vigilant but proactive in identifying and rectifying biases
Real-World Applications of Autonomous AI
The journey of autonomous AI from theoretical discussion to practical application is profound. Today, we see these systems transforming industries and impacting daily life. Let’s explore some real-world applications, focusing on their ethical implications and the responsibilities they bring.
- Healthcare: Robotic surgical systems, such as the da Vinci Surgical System, offer precision and efficiency in operations. However, questions about the extent of reliance on machines arise. When these systems malfunction, it’s critical to evaluate how human oversight can be integrated to prevent adverse outcomes.
- Transportation: Self-driving cars promise safer roads, yet they come with questions of liability and moral decisions in crisis scenarios. When faced with a potential accident, will the software prioritize the safety of passengers or bystanders? There’s a palpable tension between technological capabilities and ethical decisions, warranting robust discussions and deliberate policymaking.
- Manufacturing: Factories employing autonomous robots enhance productivity, but they also risk job displacement for many workers. This necessitates a dialogue on the ethical implications of automation in labor markets and the potential need for reskilling efforts.
- Security: Firearms equipped with AI target recognition can reduce human error in defense. Yet the prospect of machines making life-or-death decisions raises grave moral questions regarding their use in conflict or law enforcement.
Impact of AI on Employment and Labor
The tectonic shifts brought about by artificial intelligence (AI) have raised significant questions about employment and labor markets. The notable tension between technological advancement and job retention is a focal point in discussions surrounding AI. As machines take over repetitive tasks, the conversation naturally veers towards the implications for the workforce. Understanding this impact is essential for predicting shifts in employment landscapes and can help in formulating strategies to adapt.
Automation and Job Displacement
The rapid evolution of AI has catalyzed significant automation across various industries. Automated processess like self-checkout machines in grocery stores and AI-driven chatbots in customer service are just the tip of the iceberg. In many instances, companies utilize AI to reduce expenses and boost efficiency. The trade-off, however, is often job displacement. For instance, sectors such as manufacturing have seen a decline in traditional roles as robots increasingly assume assembly line tasks. While the economic benefits can be significant, the societal costs present challenges.
Considerations regarding job displacement include:
- Economic Inequality: As job sectors shrink, those without technical skills might find it increasingly difficult to secure comparable positions.
- Skill Gap: The jobs created often require a different skill set, leading to a mismatch between available talent and labor market demands.
- Mental Health Impact: The psychological toll of job loss—be it through economic uncertainty or shifting identities away from work—cannot be overlooked.
The balance between productivity gains from automation and the negative effects on workers creates a complex landscape. Economic theories suggest that while automation may lead to short-term job loss, it can also drive growth in other areas. However, this happens slowly and unevenly, often leaving the most vulnerable populations in the lurch.
Opportunities for Reskilling and Upskilling
While the displacement effects of AI are evident, one must also consider the silver lining—opportunities for reskilling and upskilling. The demand for workers who can manage, develop, and maintain AI systems is growing. Industries are starting to pivot, investing in training programs designed to equip their workers, giving a nod to the importance of human capital in the AI era.
Opportunities for growth include:
- Technical Training: As AI becomes integrated, skills in programming, data analysis, and machine learning become essential.
- Soft Skills Development: Alongside technical expertise, interpersonal skills, adaptability, and emotional intelligence are invaluable as they cannot be easily replaced by machines.
- Collaboration Between Sectors: Partnerships between educational institutions, private sector companies, and governments can yield effective reskilling programs that address local labor market needs.
"Lifelong learning is no longer a luxury but a necessity in the age of AI."
The Role of Government and Policy Makers
As artificial intelligence becomes an entrenched part of our daily lives, the role of governments and policymakers cannot be understated. They carry the significant charge of ensuring that AI technologies develop in a manner that not only fosters innovation but also addresses ethical and societal concerns. The importance lies in balancing the benefits of AI advancements with the potential risks that these technologies may pose to society—including issues like data privacy, bias, and accountability.
Regulations surrounding AI are still in their infancy. A foundation built on policy can serve as a guideline for ethical development, showing that even in fast-moving sectors, there is room for thoughtful governance. Without solid policies, companies might operate in a gray area, leading to ethical lapses that can not only damage reputations but can also harm individuals and communities.
Formulating Effective AI Policies
Formulating effective AI policies requires a multi-faceted approach, taking into consideration diverse perspectives from stakeholders. This involves:
- Consultation with Experts: Engaging with technologists, ethicists, and community representatives will provide a comprehensive outlook. Each group brings its own unique concerns, experiences, and insights, which can enrich policy considerations.
- Dynamic Frameworks: Adopt flexible policies that evolve as technology progresses. AI is not a static field; what may be ethical today could change as capabilities advance. A rigid policy might hinder progress or fail to address unforeseen issues.
- Public Input: Involving the public in discussion can lead to policies that reflect societal values and wishes. Holding town hall meetings or online forums to gather feedback can integrate the community's voice into policy formation.
- Data-Driven Decision Making: Countries should utilize existing AI data to shape their policies. This approach can help identify trends, issues, and areas requiring immediate intervention. Collecting and analyzing substantial data can lead to informed, evidence-based regulations.
Effective AI policies establish a necessary balance, guiding the ethical use of technology while encouraging innovation and growth.
International Cooperation on AI Governance
In our interconnected world, individual nations cannot address AI issues in isolation. International cooperation on AI governance can result in shared standards that ensure the responsible development and deployment of AI technologies globally. This collective approach can:
- Standardize Best Practices: Nations can learn from one another, leading to the sharing of successful frameworks and strategies. What might work in one country could provide a template for another, benefiting all involved parties.
- Address Global Challenges: Many AI issues, such as data privacy or cybersecurity threats, extend beyond borders. International frameworks can foster collaboration and collective responses to these challenges, ensuring that no country falls prey to malicious uses of AI.
- Strengthen Global Trade: Standardized regulations can enhance global trade involving AI technologies. When countries agree on rules and guidelines, it encourages businesses to operate transnationally, creating a more robust economic ecosystem.
- Promote Research and Development: By aligning efforts and pooling resources, countries can bolster their research facilities, sharing findings that benefit the entire globe's approach to AI.
As the landscape of AI continues to evolve, it’s clear that the role of government and policymakers must adapt to ensure ethical use, safety, and innovation in the digital era.
Public Perception and Ethical Discourse
Public perception serves as the backbone of how artificial intelligence is viewed in society today. It is not merely an afterthought; it's a critical pillar influencing policy-making, technological advancements, and public trust in AI systems. As AI technologies increasingly permeate daily life, understanding how the public perceives these advances and the associated ethical dilemmas becomes paramount.
A well-informed public can advocate for responsible AI usage while holding companies and governments accountable. Conversely, misinformation can breed fear, misunderstanding, and resistance to essential advancements. The influence of public perception can shape legislative actions, steer the direction of research, and ultimately define how technology impacts society.
Influence of Media on AI Perceptions
The media plays a key role in shaping public perceptions regarding AI technologies. Sensational headlines often create narratives that either exaggerate the capabilities or disparage the consequences of AI, clouding the public’s understanding. For instance, when a breakthrough in natural language processing is reported, it might lead people to believe in imminent human-like AI, sparking fear around job security and ethical concerns.
On the other hand, positive stories highlighting successful AI applications in healthcare or education can foster trust and excitement. The win-or-lose binary approach in media coverage often leads to polarized views among the audience. Here are some important elements to consider about media influence:
- Framing: How a story is presented can heavily influence its reception. A piece focusing on the potential dangers of AI may sway public opinion towards fear, while a piece showcasing AI's benefits can elicit enthusiasm.
- Repetition: Frequent exposure to certain narratives can normalize specific perceptions. For example, if reports continually associate AI with job loss, public resistance amplifies over time.
- Social Media: Platforms like Facebook and Reddit contribute significantly to disseminating information—both accurate and misleading. Misinformation can spread like wildfire, affecting how communities perceive AI.
Encouraging Public Engagement in AI Ethics
Promoting ethical discourse around AI among the general population is a responsibility that cannot be ignored. By actively engaging the public, we can demystify AI and instill a deeper understanding of its ethical implications. Here are a few strategies that can elevate public engagement:
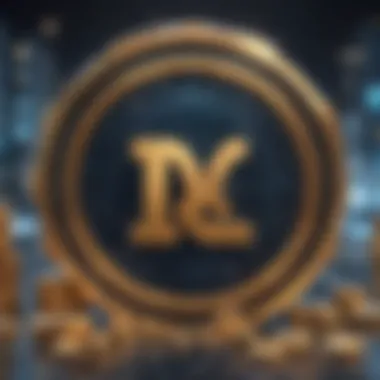
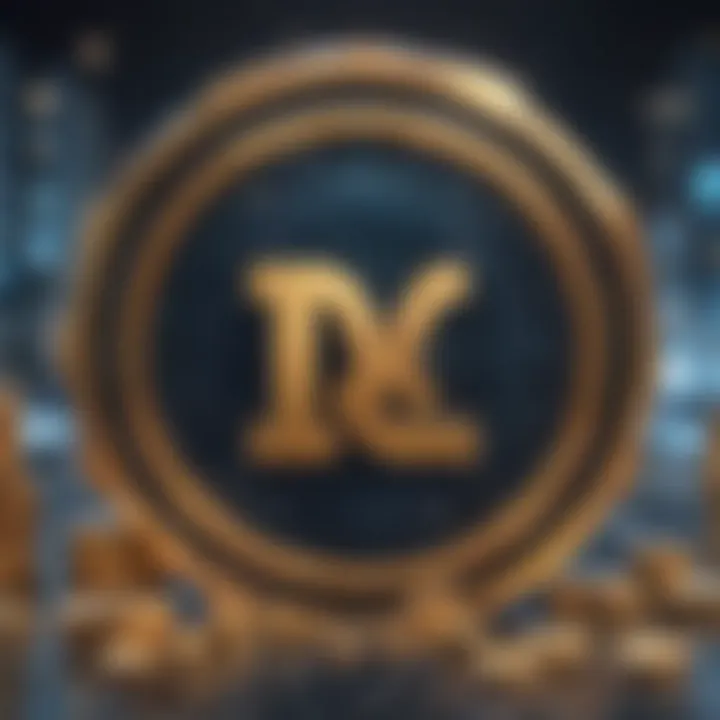
- Educational Initiatives: Workshops, webinars, and school programs can introduce AI concepts and ethical considerations early on. Education is a powerful tool for combating misinformation.
- Public Forums: Creating safe spaces for dialogue where various stakeholders can share their perspectives enables diverse input on AI policies. Gathering insights from citizens can enhance the robustness of AI ethical frameworks.
- Collaborative Research: Partnering with community organizations to conduct research on public attitudes towards AI can inform policymakers and technologists about societal expectations, fears, or hopes.
"Engaging the public in AI ethics not only empowers individuals but opens the floor to a broader range of discussions that enhance the development of responsible AI systems."
In a world increasingly shaped by AI, fostering a culture of transparency, education, and public discourse remains crucial. As society grapples with these evolving technologies, addressing public perception and ethical discourse will better prepare us for the challenges ahead.
AI in Healthcare: Ethical Challenges
The integration of artificial intelligence into the healthcare sector offers both groundbreaking potential and intricate ethical challenges. This intersection is crucial as it significantly impacts patient care, medical treatments, and even health policy. It’s not merely about harnessing technology to provide faster diagnostics or personalized medicine; it also evokes significant questions surrounding ethical standards, equity, and the fundamental human rights tied to healthcare delivery.
Healthcare professionals now have access to increasingly sophisticated AI tools that can analyze data faster than any human specialist. The benefits are numerous: enhanced diagnostic accuracy, predictive analytics for disease outbreaks, and operational efficiencies that can transform patient care dynamics. Yet, this rapid incorporation of AI dredges up concerns over accountability, data privacy, and the transparency of decision-making processes.
Given such stakes, understanding the balance between innovation and ethical practice is paramount. Ethical scrutiny in AI healthcare applications ensures fundamental considerations like patient autonomy, informed consent, and the avoidance of bias remain front and center.
"As we embrace AI in healthcare, we must ensure that ethical guidelines keep pace with technological advancements."
Use of AI in Medical Diagnosis
AI's role in medical diagnosis is perhaps one of the most compelling aspects of its application in healthcare. Algorithms now assist doctors in identifying diseases at earlier stages than ever before. For example, AI systems can analyze medical images, such as X-rays or MRIs, with precision that sometimes surpasses human detection. This capability does not just aim for efficiency; it can be a matter of life and death in terms of treatment decisions.
However, the integration of AI also requires a thorough investigation into how these systems make decisions. If the rationale behind an AI-generated diagnosis is murky, clinicians and patients may have difficulty trusting its accuracy. Thus, it becomes vital to demand transparency in these processes. Clinicians must understand how AI arrives at specific conclusions so they can confidently provide appropriate care based on its recommendations.
Patient Consent and Data Usage
In the age of digital health records and telemedicine, understanding patient consent is more important than ever. Patient data fuels the algorithms that drive AI innovations. So, is it enough merely to inform patients that their data might contribute to research or diagnostic improvements? Or should we strive for an even more nuanced form of engagement that allows patients greater control over how their information is used?
Informed consent is not just a legal requirement; it is an ethical imperative. Patients ought to comprehend the potential uses of their data, including how long it will be stored, who will access it, and what safeguards are in place to protect their privacy. Failure to prioritize clear and proactive consent policies risks undermining public trust in health systems deploying AI.
Ultimately, the future of AI in healthcare depends not only on the technology itself but also on the ethical framework we establish around its use. This balancing act must ensure that the benefits of AI do not come at the expense of fundamental human rights.
The Future of AI and Ethical Considerations
The landscape of artificial intelligence is shifting rapidly, making the exploration of its future particularly urgent. As we look towards a digital age characterized by unprecedented advancements in AI, understanding ethical considerations becomes not only important but necessary. This section aims to illuminate several facets pertaining to the ethical dimensions of AI’s evolution, as well as the significance of choosing an ethical path forward.
What is at stake includes the welfare of individuals and societies alike. As AI systems become increasingly embedded in our daily lives—from healthcare delivery systems to financial decision-making tools—the ethical implications of these technologies grow quantitatively and qualitatively. Emphasizing ethics isn’t just about avoiding harm; it’s about maximizing benefits for everyone. Striking a balance between innovation and safeguarding human values is key. It’s not merely an academic exercise; the choices we make today will reverberate across generations.
Emerging Trends in AI Ethics
In the rapidly changing world of AI, certain trends have become apparent, shaping the ethical discourse significantly. One prominent trend is the emphasis on collaborative ethics. This approach advocates for involving diverse stakeholders in the development of AI systems, ensuring multiple perspectives are considered. It’s like assembling a choir: each voice contributes to a richer harmony.
Another developing trend is the call for more robust regulatory frameworks that govern AI technologies. As AI becomes more powerful, there are growing demands for regulations that not only prevent misuse but also foster accountability. Regulations that emphasize transparency and fairness can serve as the foundation for trust in these technologies.
A third trend relates to the utilization of explainable AI. Users should have insight into how AI systems reach decisions, especially in critical areas like healthcare or criminal justice. This isn’t just about compliance; it’s about building confidence and credibility in AI applications.
- Collaborative Ethics: Involvement of multiple stakeholders in AI development.
- Robust Regulatory Frameworks: Creating laws that not only prevent misuse but also encourage accountability.
- Explainable AI: Ensuring transparency in decision-making processes of AI systems.
Preparing for Ethical Challenges Ahead
As AI becomes a staple in various sectors, the anticipation of ethical challenges may seem daunting, but proactive strategies can ease this transition. One effective strategy is education and awareness. By developing comprehensive education programs that highlight the ethical implications of AI technologies, stakeholders—from developers to end-users—can better understand their responsibilities and societal impacts.
Moreover, organizations should establish ethics review boards to oversee the development and implementation of AI systems. These boards can act as a safeguard against potential ethical breaches that might arise. Like having a ship captain consult their navigational charts, these review boards can help steer technologies in a more ethical direction.
In addition, leveraging AI ethics frameworks can guide organizations in structuring their approach to ethical dilemmas. Such frameworks can act as a compass in complex scenarios, ensuring that considerations of benefit, harm, fairness, and accountability are integral to the decision-making process.
As articulated in a report from the World Economic Forum, ethical considerations in AI are not optional; rather, they are prerequisites for sustainable innovation.
In summary, navigating the future of AI requires a concerted effort toward ethical understanding and action. With emerging trends steering discussions on collaborative efforts, regulatory frameworks, and explainability, it becomes clear that planning for ethical challenges ahead is a necessary commitment. Balancing advancement with ethical integrity will not only cultivate innovation but also fortify the very foundation of societal trust in AI technologies.
Case Studies of Ethical Dilemmas in AI
Case studies of ethical dilemmas in artificial intelligence are crucial. They help us understand how AI technologies are implemented and the convoluted ethical landscapes those implementations create. Observing real-world situations where ethical principles are challenged offers invaluable lessons. It makes the abstract discussions of ethics more tangible. Moreover, these cases can illuminate potential pitfalls, guiding developers and policymakers in crafting better systems that consider ethical implications. By analyzing these scenarios, we gain insight into the consequences of AI decisions and the far-reaching impacts on individuals and society at large.
Notable Examples from Various Industries
- Healthcare: One notable instance occurred when an AI-based diagnostic tool was developed to prioritize patients needing urgent attention. While the tool was efficient, it raised concerns about potential biases in patient data that could lead to discrimination against certain groups. If the training data didn't adequately represent diversity, the AI might misidentify healthcare needs.
- Finance: In the financial sector, algorithmic trading systems have faced scrutiny. For example, a trading algorithm inadvertently caused a stock market crash due to its reliance on historical patterns without accounting for current market conditions. It highlighted the risks in over-dependence on AI in high-stakes environments.
- Autonomous Vehicles: A well-publicized case involved an autonomous vehicle that struck a pedestrian. Investigations revealed that the AI lacked the necessary data to distinguish between a jaywalking pedestrian and a stationary object. This incident raised questions about accountability. Should the algorithm, the developers, or the vehicle's owner be held responsible?
- Social Media Platforms: Several platforms use AI to control content distribution. A significant example was when an AI moderation system flagged misinformation. However, it led to the suppression of legitimate posts, demonstrating that while AI can improve user experience, it can also stifle free speech if not managed properly.
Lessons Learned from Each Case
- Understanding Bias: The healthcare case underscores the importance of inclusive datasets. Without diversity in data, AI can perpetuate existing biases, highlighting the need for ongoing reviews and updates in training data.
- Caution with Automation: The financial trading example teaches the lesson of caution. Even sophisticated algorithms can miscalculate; thus, there's a need for human oversight, especially in volatile markets.
- Accountability Structures: The tragic accident involving the autonomous vehicle points to a pressing need for clear accountability standards. Defining responsibility can deter negligence in AI development and deployment.
- Transparent Content Moderation: The social media example illustrates the necessity of transparency regarding AI policies and moderation decisions. Users should be aware of how their content is evaluated, fostering trust and comprehension.
"These case studies are not just anecdotes; they're pointers that inform how we navigate the complex terrain of AI ethics."
Through these real-life examples, we can reflect on key ethical dilemmas that surface across various industries. Grappling with these lessons will lead to more nuanced approaches in the future, ensuring AI serves humanity better while upholding ethical standards.
Concluding Thoughts on AI and Ethics
As we wrap up our exploration of the complex landscape of artificial intelligence and its ethical implications, it becomes clear that this subject is no mere sideshow in the digital age. Rather, it stands as a cornerstone of contemporary discourse and practice. The integration of AI into various sectors not only redefines operational frameworks but also brings to the fore critical ethical questions that every stakeholder must grapple with.
The importance of concluding thoughts on AI ethics lies in the acknowledgment that technology is a tool shaped by human intentions. With each deployment of an AI system, whether in healthcare, finance, or transportation, we invite both potential breakthroughs and unforeseen challenges. Therefore, understanding the implications of our choices becomes imperative.
The key benefits of addressing AI ethics include:
- Promoting trust: As AI systems increasingly influence daily life, transparent ethical frameworks generate trust among users, ensuring they feel secure in relying on these technologies.
- Encouraging innovation: Ethical considerations can often act as a springboard for new ideas. By addressing potential pitfalls, innovators can create solutions that are not just effective but also socially responsible.
- Safeguarding values: A well-articulated ethical commitment ensures that the deployment of AI systems aligns with societal values, thus avoiding the pitfalls of bias and discrimination.
Thus, while AI serves to enhance efficiency and capabilities across sectors, it also necessitates vigilance against ethical lapses. By navigating the delicate balance between progress and principle, stakeholders can ensure that artificial intelligence serves humanity rather than undermines it.
The Path Forward for AI Ethics
Looking ahead, it's essential to craft a roadmap for the ethical landscape of AI. The next steps should involve a multi-faceted approach:
- Research and Education: Foster a culture of continuous learning both in academia and within industries. Professionals should be well-versed in ethical implications to make informed decisions about AI implementations.
- Robust Policies: Governments and organizations must draft clear policies that address ethical concerns up front. This encourages a proactive rather than reactive attitude towards ethical dilemmas.
- Community Engagement: Encourage broader community participation in discussions about AI ethics. Ensuring diverse voices contribute to the conversation will deliver more robust solutions.
- Continuous Evaluation: The impact of AI should be continuously assessed, adjusting policies and practices as necessary to meet evolving ethical standards.
By laying down these foundations, society can harness the benefits of artificial intelligence while steering clear of the ethical pitfalls that can arise from its misuse.
The Importance of Ongoing Discourse
For ethics in AI to remain relevant, ongoing discourse is crucial. Without continued discussion and evaluation, it’s easy to slip into complacency. Here's why periodic reflection is essential:
- Adaptation to Change: The rapid evolution of technology means that ethical frameworks must adapt to new challenges. Ongoing discourse captures shifting paradigms and societal norms.
- Enhanced Awareness: Regular conversations raise awareness of ethical issues. Informed advocates can better argue for necessary changes, whether in corporate boardrooms or legislative halls.
- Inclusive Perspectives: Engaging diverse stakeholders ensures that the ethical considerations reflect a multitude of viewpoints, helping to identify blind spots that could lead to misuse or misunderstanding of AI technologies.
By committing to vibrant and ongoing discussions around AI ethics, we can ensure that both innovation and responsibility flourish. As we navigate this digital age, prioritizing ethical considerations serves as our compass, guiding us toward a future where artificial intelligence respects and upholds human dignity.